Social Psychology and Society
2024. Vol. 15, no. 2, 117–139
doi:10.17759/sps.2024150208
ISSN: 2221-1527 / 2311-7052 (online)
Sex or Smartphone? – Analysis of the Relationship between Problematic Smartphone Usage and Sexual Activity Based on Homogeneous and Heterogeneous IDs and Machine Learning Algorithms
Abstract
Objective. Our study explores the correlation between problematic smartphone use (PSU) and diminished offline sexual activity within a European Union member state characterized by a semi-peripheral economy. Background. Smartphones, as pervasive technological advancements, have transformed societal landscapes, embedding themselves into various facets of life and exacerbating physical and emotional reliance. Over 50% of users continue smartphone use despite adverse effects on daily life, indicating an escalation in PSU. Our research extends existing PSU literature by investigating its relationship with offline sexual inactivity among middle-aged individuals.
Study Design. A representative sample from 2023 was analyzed using both homogeneous (Two-NN) and heterogeneous (HIDALGO) dimensional identification estimators alongside machine learning algorithms to explore the link between PSU and offline sexual inactivity. Participants. The study utilized data from a telephone survey conducted with 1005 individuals, ensuring representation across gender, education, income level, and type of settlement.
Measurements. Data encompassed economic, sociodemographic, usage patterns, and addiction-related aspects of smartphone use. A key variable assessed preferences between mobile phone use or engaging in sexual intercourse.
Results. Nearly half of the participants expressed a preference for smartphone usage over offline sexual activity. The analysis highlighted the intricate link between individual and social aspects of PSU and a blend of socioeconomic factors, revealing two significant partitions significantly influencing sexual inactivity: PSU at the individual level and PSU articulated within social relationships.
Conclusions. Our findings indicate a significant correlation between PSU and offline sexual inactivity, with socioeconomic variables also playing a critical role. The research underscores the need for further exploration of PSU's impact on offline sexual activity, emphasizing the importance of both personal and social psychological dimensions of smartphone usage.
General Information
Keywords: problematic smartphone use; sexual activity; homogeneous and heterogeneous estimator; machine learning
Journal rubric: Empirical Research
Article type: scientific article
DOI: https://doi.org/10.17759/sps.2024150208
Received 31.01.2024
Accepted
Published
For citation: Gosztonyi, M. (2024). Sex or Smartphone? – Analysis of the Relationship between Problematic Smartphone Usage and Sexual Activity Based on Homogeneous and Heterogeneous IDs and Machine Learning Algorithms. Social Psychology and Society, 15(2), 117–139. https://doi.org/10.17759/sps.2024150208
© Gosztonyi M., 2024
License: CC BY-NC 4.0
Full text
Introduction
Sex Or Phone |
Description of the variable’s categories |
Frequency |
Frequency Percentage |
No Sex |
I would rather give up sex for 12 months just to use my smartphone |
401 |
44,53% |
No Phone |
I would rather give up my smartphone, but I can't live without sex for 12 months |
500 |
55,47% |
Total |
901 |
100% |
Methodology
Results
Variable |
Mean |
Std Dev |
Min |
25% |
50% |
75% |
Max |
Use Mobile Phone |
0,993 |
0,083 |
0 |
1 |
1 |
1 |
1 |
How Long Use Smartphone |
7,616 |
1,081 |
1 |
8 |
8 |
8 |
8 |
How Often Download Apps |
1,351 |
0,928 |
0 |
1 |
1 |
2 |
4 |
Use Apps for Socialmedia |
0,770 |
0,421 |
0 |
1 |
1 |
1 |
1 |
Household Size |
2,626 |
1,114 |
1 |
2 |
2 |
3 |
7 |
Monthly Net Income |
5,500 |
1,882 |
1 |
5 |
5 |
6 |
12 |
Education Categhory |
3,286 |
0,800 |
1 |
3 |
3 |
4 |
4 |
Sex (Male, Female) |
1,542 |
0,498 |
1 |
1 |
2 |
2 |
2 |
Sex Or Phone |
1,599 |
0,490 |
1 |
1 |
2 |
2 |
2 |
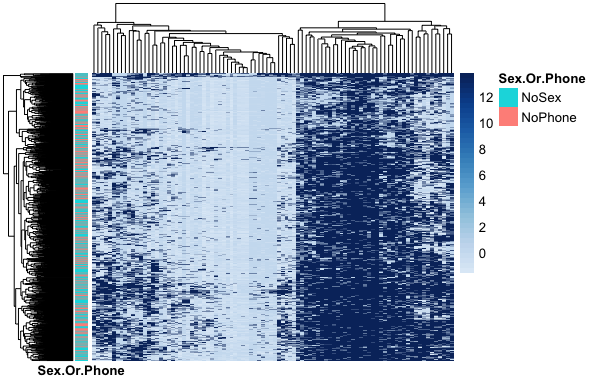
Analysis |
Lower_Bound |
Estimate |
Upper_Bound |
Mean |
Median |
Mode |
Linfit |
22,498 |
22,640 |
22,889 |
|
|
|
Bayes |
22,503 |
NE |
25,662 |
24,057 |
24,048 |
24,030 |
MLE |
22,504 |
24,031 |
25,663 |
|
|
|
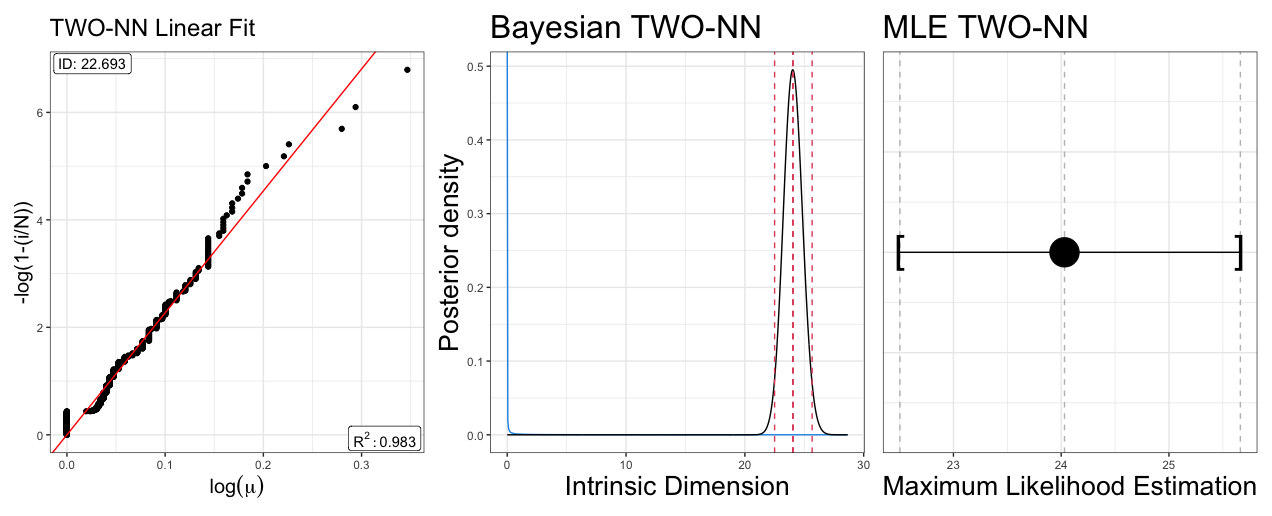
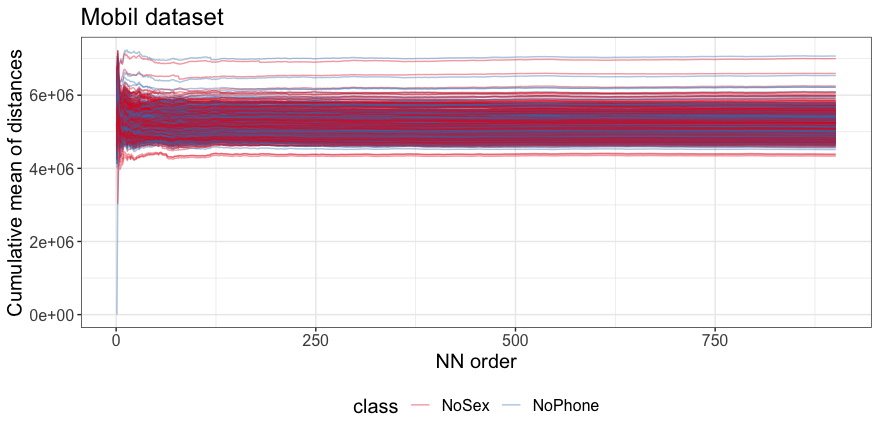
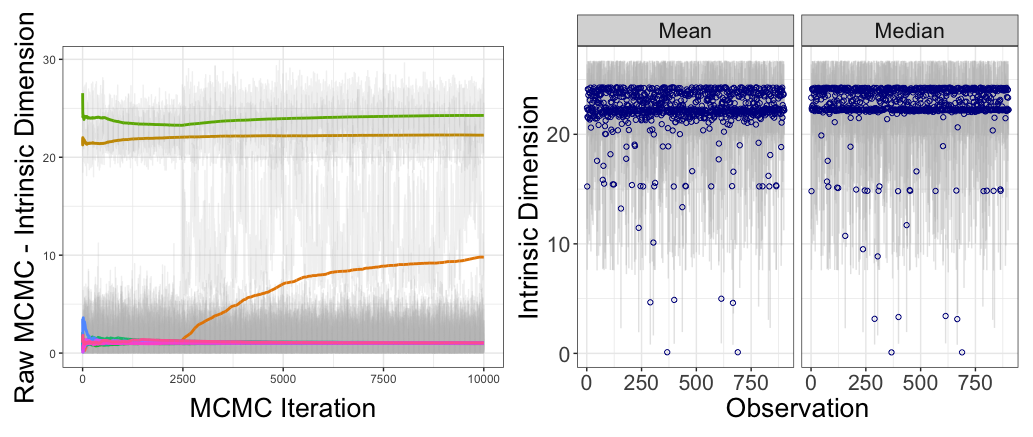
class |
mean |
median |
sd |
No Sex |
23,177 |
23,027 |
2,634 |
No Phone |
23,363 |
23,064 |
2,559 |
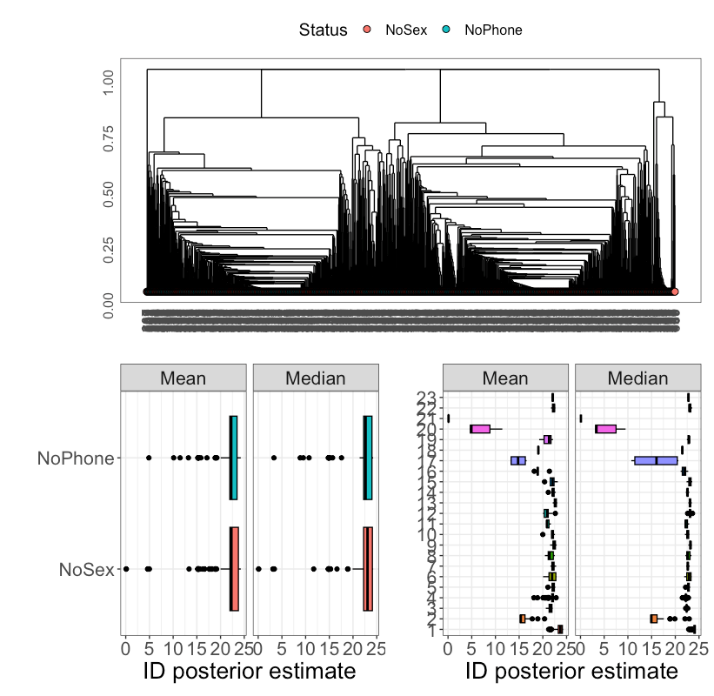
Values |
Precision |
Recall sensitivity |
F1 score |
No Sex |
0,803 |
0,874 |
0,837 |
No Phone |
0,875 |
0,955 |
0,912 |
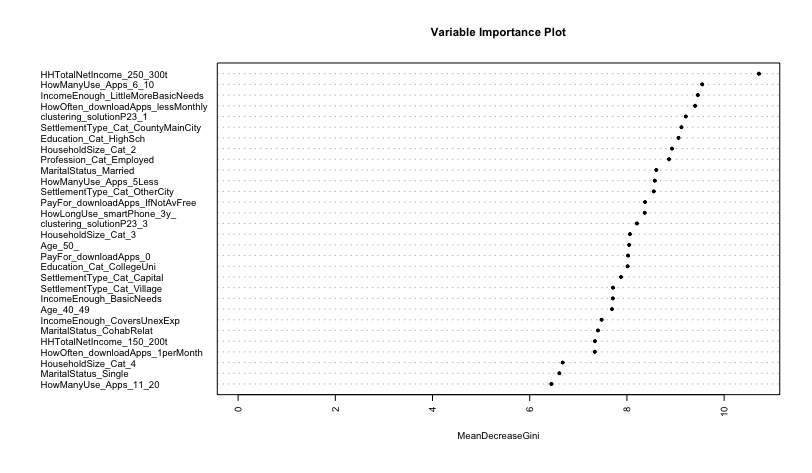
Discussion
Conclusion
Cluster No |
No Sex |
No Phone |
Proportion of No Phone |
Average posterior ID mean |
Average posterior ID median |
Average posterior ID sd |
|
||
P2 Cluster structure |
|
||||||||
1 |
496 |
403 |
0,448 |
22,333 |
22,261 |
2,090 |
|
||
2 |
2 |
0 |
0,000 |
0,104 |
0,104 |
0,000 |
|
||
P3 Cluster structure |
|
||||||||
1 |
2 |
0 |
0,000 |
0,104 |
0,104 |
0,000 |
|
||
2 |
206 |
200 |
0,493 |
2,957 |
22,074 |
0,477 |
|
||
3 |
290 |
203 |
0,412 |
22,643 |
23,549 |
2,753 |
|
||
P4 Cluster structure |
|
||||||||
1 |
213 |
202 |
0,487 |
23,109 |
23,047 |
0,459 |
|
||
2 |
133 |
108 |
0,448 |
25,977 |
26,348 |
0,906 |
|
||
3 |
147 |
90 |
0,380 |
21,396 |
21,302 |
0,931 |
|
||
4 |
5 |
3 |
0,375 |
4,528 |
1,775 |
5,106 |
|
||
P23 Cluster structure |
|
||||||||
1 |
232 |
163 |
0,413 |
23,587 |
23,710 |
0,639 |
|
||
2 |
18 |
9 |
0,333 |
16,034 |
15,342 |
1,346 |
|
||
3 |
3 |
3 |
0,500 |
21,513 |
21,560 |
0,538 |
|
||
4 |
168 |
165 |
0,496 |
21,955 |
22,069 |
0,450 |
|
||
5 |
14 |
13 |
0,482 |
22,115 |
22,182 |
0,399 |
|
||
6 |
4 |
4 |
0,500 |
21,854 |
21,951 |
0,997 |
|
||
7 |
4 |
8 |
0,667 |
22,123 |
22,144 |
0,345 |
|
||
8 |
4 |
2 |
0,333 |
21,553 |
21,447 |
0,810 |
|||
9 |
7 |
7 |
0,500 |
22,332 |
22,410 |
0,411 |
|||
10 |
6 |
0 |
0,000 |
21,776 |
22,002 |
0,913 |
|||
11 |
2 |
1 |
0,333 |
21,106 |
20,863 |
0,527 |
|||
12 |
8 |
3 |
0,273 |
20,914 |
20,893 |
0,823 |
|||
13 |
6 |
6 |
0,500 |
22,588 |
22,584 |
0,306 |
|||
14 |
4 |
5 |
0,556 |
22,070 |
22,078 |
0,450 |
|||
15 |
1 |
3 |
0,750 |
21,899 |
22,036 |
1,154 |
|||
16 |
4 |
1 |
0,200 |
19,262 |
18,901 |
1,229 |
|||
17 |
3 |
1 |
0,250 |
14,843 |
14,781 |
1,802 |
|||
18 |
1 |
2 |
0,667 |
19,035 |
19,060 |
0,136 |
|||
19 |
2 |
1 |
0,333 |
20,894 |
21,320 |
1,502 |
|||
20 |
3 |
3 |
0,500 |
6,787 |
4,936 |
3,132 |
|||
21 |
2 |
0 |
0,500 |
0,104 |
0,104 |
0,000 |
|||
22 |
1 |
2 |
0,667 |
22,219 |
22,332 |
0,458 |
|||
23 |
1 |
1 |
0,500 |
22,083 |
22,083 |
0,244 |
|||
References
- Ali N., Neagu D., Trundle P. Evaluation of k-nearest neighbour classifier performance for heterogeneous data sets. SN Applied Sciences, 2019. Vol. 1, pp. 1–15.
- Aljomaa S.S., Qudah M.F.Al, Albursan I.S., Bakhiet S.F., Abduljabbar A.S. Smartphone addiction among university students in the light of some variables. Computers in Human Behavior, 2016. Vol. 61, pp. 155–164.
- Allegra M., Facco E., Denti F., Laio A., Mira A. Data Segmentation Based on the Local Intrinsic Dimension. Scientific Reports, 2020. Vol. 10, no. 1, pp. 32–49.
- Amerio A., Lugo A., Bosetti C., Fanucchi T., Gorini G., Pacifici R., Gallus S. Italians Do it… less. COVID-19 lockdown impact on sexual activity: Evidence from a large representative sample of Italian adults. Journal of Epidemiology, 2021. Vol. 31, no. 12, pp. 648–652.
- Ansuini A., Laio A., Macke J.H., Zoccolan D. Intrinsic Dimension of Data Representations in Deep Neural Networks. In Proceedings of the 33rd International Conference on Neural Information Processing Systems, 2019. pp. 6111–6122.
- Ayar D., Bektas M., Bektas I., Kudubes A.A., Ok Y.S., Altan S.S. et al. The effect of adolescents’ internet addiction on smartphone addiction. Journal of Addictions Nursing, 2017. 28, no. 4, pp. 210–214.
- Bae S.M. The relationship between the type of smartphone use and smartphone dependence of Korean adolescents: National survey study. Children and Youth Services Review, 2017. Vol. 81, no. 1, pp. 207–211.
- Barnes S.J., Pressey A.D., Scornavacca E. Mobile ubiquity: Understanding the relationship between cognitive absorption, smartphone addiction and social network services. Computers in Human Behavior, 2019. Vol. 90, pp. 246–258.
- Benotsch E.G., Snipes D.J., Martin A.M., Bull S.S. Sexting, substance use, and sexual risk behavior in young adults. Journal of Adolescent Health, 2012. Vol. 38, no. 1, pp. 8–12.
- Beutel M.E., Burghardt J., Tibubos A.N., Klein E.M., Schmutzer G., Brähler E. Declining sexual activity and desire in men: findings from representative German surveys, 2005 and 2016. Journal of Sexual Medicine, 2018. Vol. 15, no. 5, pp. 750–756. DOI:10.1016/j.jsxm.2018.03.010
- Billieux J. Problematic use of the mobile phone: A literature review and a pathways model. Current Psychiatry Reviews, 2012. Vol. 8, no. 4, pp. 299–307.
- Bodenmann G., Atkins D.C., Schär M., Poffet V. The association between daily stress and sexual activity. Journal of Family Psychology, Vol. 24, no. 3, pp. 271–279. DOI:10.1037/a0019365
- Boulesteix A.L., Janitza S., Kruppa J., König I.R. Overview of random forest methodology and practical guidance with emphasis on computational biology and bioinformatics. Wiley Interdisciplinary Reviews: Data Mining and Knowledge Discovery, 2012. Vol. 2, no. 6, pp. 493–507.
- Brand M., Wegmann E., Stark R., Müller A., Wölfling K., Robbins T.W., Potenza M.N. The Interaction of Person-Affect-Cognition-Execution (I-PACE) model for addictive behaviors: Update, generalization to addictive behaviors beyond internet-use disorders, and specification of the process character of addictive behaviors. Neuroscience & Biobehavioral Reviews, 2019. Vol. 104, pp. 1–10.
- Breiman L. Classification and regression trees. London: Routledge, 2017. 368 p.
- Carmichael M.S., Humbert R., Dixen J., Palmisano G., Greenleaf W., Davidson J.M. Plasma oxytocin increases in the human sexual response. The Journal of Clinical Endocrinology & Metabolism, 1987. Vol. 64, no. 1, pp. 27–
- Chavent M., Kuentz V., Liquet B., Saracco L. ClustOfVar: An R package for the clustering of variables. arXiv preprint arXiv:1112.0295. London: Routledge, DOI:10.48550/arXiv.1112.0295
- Chen L., Yan Z., Tang W., Yang F., Xie X., He J. Mobile phone addiction levels and negative emotions among Chinese young adults: The mediating role of interpersonal problems. Computers in Human Behavior, 2016. Vol. 55, pp. 856–866.
- Chen I.F., Tsaur R.C., Chen P.Y. Selection of best smartphone using revised electre-iii method. International Journal of Information Technology & Decision Making, 2018. Vol. 17, pp. 1915–1936.
- Cheng L., De Vos J., Zhao P., Yang M., Witlox F. Examining non-linear built environment effects on elderly’s walking: A random forest approach. Transportation Research Part D: Transport and Environment, 2020. Vol. 88, pp. 102552–
- Chotpitayasunondh V., Douglas K.M. How “phubbing” becomes the norm: The antecedents and consequences of snubbing via smartphone. Computers in Human Behavior, 2016. Vol. 63, pp. 9–18.
- Clayton R.B., Leshner G., Almond A. The extended iSelf: The impact of iPhone separation on cognition, emotion, and physiology. Journal of Computer – Mediated Communication, 2015. Vol. 20, no. 2, pp. 119–135. DOI:10.1111/jcc4.12109
- Cohen J. A coefficient of agreement for nominal data. Educational and Psychological Measurement, 1960. Vol. 20, pp. 37–46.
- Courtice E.L., Shaughnessy K. Technology-mediated sexual interaction and relationships: A systematic review of the literature. Sexual and Relationship Therapy, 2017. Vol. 32, no. 3-4, pp. 269–
- Denti F. intRinsic: an R package for model-based estimation of the intrinsic dimension of a dataset. arXiv preprint arXiv:2102.11425. London: Routledge, 2021.DOI:48550/ARXIV.2102.11425
- De-Sola J., Talledo H., Rubio G., de Fonseca F.R. Psychological factors and alcohol use in problematic mobile phone use in the Spanish population. Frontiers in Psychiatry, 2017. Vol. 8, pp. 11– DOI:10.3389/fpsyt.2016.00175
- Dodaj A., Sesar K. Sexting categories. Mediterranean Journal of Clinical Psychology, 2020. Vol. 8, no. 2, pp. 1–
- Dupree J.M., Langille G.M. The Impact of the Environment on Sexual Health. In: Lipshultz L., Pastuszak A., Goldstein A., Giraldi A., Perelman M. (eds.). Management of Sexual Dysfunction in Men and Women. New York, NY: Springer, 2016, pp. 17–24.
- Eichenberg C., Schott M., Schroiff A. Comparison of students with and without problematic smartphone use in light of attachment style. Frontiers in Psychiatry, 2019. Vol. 10, pp. 681– DOI:10.3389/fpsyt.2019.00681
- Eleuteri S., Saladino V., Verrastro V. Identity, relationships, sexuality, and risky behaviors of adolescents in the context of social media. Sexual and Relationship Therapy, 2017. Vol. 32, no. 3, pp. 1–12. DOI:1080/14681994.2017.1397953
- Elhai J.D., Levine J.C., Dvorak R.D., Hall B.J. Fear of missing out, need for touch, anxiety, and depression are related to problematic smartphone use. Computers in Human Behavior, 2016. Vol. 63, pp. 509–516. DOI:1016/j.chb.2016.05.079
- Engeström Y. Innovative learning in work teams: Analyzing cycles of knowledge creation in practice. In Y. Engeström, R. Miettinen & R.-L. Punamäki (Eds.). Perspectives on Activity Theory. Learning in doing: Social, cognitive, and computational perspectives. Cambridge: Cambridge University Press, 1999, pp. 377–404.
- Ferguson C.J. Sexting behaviors among young Hispanic women: Incidence and association with other high-risk sexual behaviors. Psychiatric Quarterly, 2011. Vol. 82, pp. 239–243.
- Ferrante L., Venuleo C. Problematic Internet Use among adolescents and young adults: a systematic review of scholars’ conceptualisations after the publication of DSM-5. Mediterranean Journal of Clinical Psychology, 2021. Vol. 9, no. 2, pp. 12–33. DOI:10.13129/2282-1619/mjcp-3016
- Gao Q., Fu E., Xiang Y., Jia G., Wu S. Self-esteem and addictive smartphone use: the mediator role of anxiety and the moderator role of self-control. Child Youth Serv Rev, Vol. 124, pp. 105990–106004.
- Goldstein B.A., Hubbard A.E., Cutler A., Barcellos L.F. An application of Random Forests to a genome-wide association dataset: methodological considerations & new findings. BMC genetics, 2010. Vol. 11, no. 1, pp. 1–
- Hao Z., Jin L. Alexithymia and problematic mobile phone use: a moderated mediation model. Frontiers in Psychology, 2020. Vol. 11, pp. 541507–
- Hartanto A., Yang H. Is the smartphone a smart choice? The effect of smartphone separation on executive functions. Computers in Human Behavior, 2016. Vol. 64, pp. 329–336.
- Harwood J., Dooley J.J., Scott A.J., Joiner R. Constantly connected–The effects of smart-devices on mental health. Computers in Human Behavior, 2014. Vol. 34, pp. 267–272. DOI:1016/j.chb.2014.02.006
- Heitjan D.F., Basu S. Distinguishing “missing at random” and “missing completely at random”. The American Statistician, 1996. 50, no. 3, pp. 207–213.
- Hong F.-Y., Chiu S.-I., Huang D.-H. A model of the relationship between psychological characteristics, mobile phone addiction and use of mobile phones. Computers in Human Behavior, Vol. 114, pp. 106414–106431.
- Hong W., Liu R.-D., Oei T.-P., Zhen R., Jiang S., Sheng X. The mediating and moderating roles of social anxiety and relatedness need satisfaction on the relationship between shyness and problematic mobile phone use among adolescents. Computers in Human Behavior, 2019. Vol. 93, pp. 301–308.
- Horwood S., Anglim J. Personality and problematic smartphone use: A facet-level analysis using the five factor model and HEXACO frameworks. Computers in Human Behavior, 2018. Vol. 85, pp. 349–359. DOI:1016/j.chb.2018.04.013
- Ihm J. Social implications of children’s smartphone addiction: The role of support networks and social engagement. Journal of Behavioral Addictions, 2018. Vol. 7, no. 2, pp. 473–481.
- Kahneman D., Krueger A.B., Schkade D., Schwarz N., Stone A. Toward national well-being accounts. American Economic Review, 2004. 94, no. 2, pp. 429–434. DOI:10.1257/0002828041301713
- Kim E., Koh E. Avoidant attachment and smartphone addiction in college students: The mediating effects of anxiety and self-esteem. Computers in Human Behavior, 2018. Vol. 84, pp. 264–271. DOI:10.1016/j.chb.2018.02.037
- Kim J.H., Tam W.S., Muennig P. Sociodemographic correlates of sexlessness among American adults and associations with self-reported happiness levels: evidence from the U.S. General Social Survey. Archives of Sexual Behavior, 2017. Vol. 46, no. 8, pp. 2403–
- Király O., Demetrovics Z. Problematic internet use. In Király O., Demetrovics Z. (Eds.). Textbook of Addiction Treatment. New York: Springer, 2021, pp. 955–965.
- Konok V., Pogany A., Miklosi A. Mobile attachment: Separation from the mobile phone induces physiological and behavioural stress and attentional bias to separation-related stimuli. Computers in Human Behavior, 2017. Vol. 71, pp. 228–239.
- Krumpal I. Determinants of social desirability bias in sensitive surveys: a literature review. Quality & quantity, 2013. Vol. 47, no. 4, pp. 2025–2047.
- Kuhn M., Johnson K. Applied predictive modeling. New York: Springer, 600 p.
- Kuhn M. Caret: Classification and Regression Training. R package version 6.0-71. Cham: R-Studio, 2016.
- Kumcagiz H., Gündüz Y. Relationship between psychological well-being and smartphone addiction of university students. International Journal of Higher Education, 2016. Vol. 5, pp. 144–156.
- Kwon M., Kim D.-J., Cho H., Yang S. The Smartphone Addiction Scale: Development and Validation of a Short Version for Adolescents. PLoS One, 2014. Vol. 8, pp. e83558–e83572.
- Kwon H.E., So H., Han S.P., Oh W. Excessive dependence on mobile social apps: A rational addiction perspective. Information Systems Research, 2016. Vol. 27, no. 4, pp. 919–939. DOI:1287/isre.2016.0658
- Lapointe L., Boudreau-Pinsonneault C., Vaghefi I. Is smartphone usage truly smart? A qualitative investigation of it addictive behaviors. In 2013 46th Hawaii international conference on system sciences, 2013, pp. 1063–1072. DOI:1109/HICSS.2013.367
- Lee G., Rodriguez C., Madabhushi A. Investigating the Efficacy of Nonlinear Dimensionality Reduction Schemes in Classifying Gene and Protein Expression Studies. IEEE/ACM Transactions on Computational Biology and Bioinformatics, 2008. Vol. 5, no. 3, pp. 368–384. DOI:10.1109/tcbb.2008.36
- Lee H., Kim J.W., Choi T.Y. Risk factors for smartphone addiction in Korean adolescents: Smartphone use patterns. Journal of Korean Medical Science, 2017. Vol. 32, no. 10, pp. 1674–1679.
- Lepp A., Li J., Barkley J.E. College students’ cell phone use and attachment to parents and peers. Computers in Human Behavior, 2016. Vol. 64, pp. 401–408.
- Li L., Trisha L. Examining how dependence on smartphones at work relates to Chinese employees ́ workplace social capital, jobperformance, and smartphone addiction. SAGE, 2017. Vol. 34, no. 5, pp. 1–14. DOI:1177/0266666917721735
- Majeur D., Leclaire S., Raymond C., Léger P.M., Juster R.P., Lupien S.J. Mobile phone use in young adults who self‐identify as being “Very stressed out” or “Zen”: An exploratory study. Stress and Health, 2020. Vol. 36, no. 5, pp. 606–614.
- Malley J.D., Malley K.G., Pajevic S. Statistical Learning for Biomedical Data. Cambridge: Cambridge University Press, 2011. 298 p.
- Mercer C.H., Tanton C., Prah P. et al. Changes in sexual attitudes and lifestyles in Britain through the life course and over time: findings from the National Surveys of Sexual Attitudes and Lifestyles (Natsal). Lancet, 2013. Vol. 382, pp. 1781–1794. DOI:10.1016/S0140-6736(13)62035-8
- Meskó N., Őry F. The functioning of the sexual system questionnaire is the Hungarian version (SSFS). Magyar Pszichológiai Szemle, 2023. Vol. 78, no. 1, pp. 113–134.
- Nahas M., Hlais S., Saberian C., Antoun J. Problematic smartphone use among Lebanese adults aged 18–65 years using MPPUS-10. Computers in Human Behavior, 2018. Vol. 87, pp. 348–353. DOI:1016/j.chb.2018.06.009
- Ueda P., Mercer C.H., Ghaznavi C., Herbenick D. Trends in frequency of sexual activity and number of sexual partners among adults aged 18 to 44 years in the US, 2000-2018. JAMA Network Open, 2020. Vol. 3, no. 6, pp. e203833–e203833.
- Panda A., Jain N.K. Compulsive smartphone usage and users’ ill-being among young Indians: Does personality matter? Telematics and Informatics, 2018. Vol. 35, no. 5, pp. 1355–1372. DOI:1016/j.tele.2018.03.006
- Parasuraman S., Sam A.T., Yee S., Chuon B., Ren L.Y. Smartphone usage and increased risk of mobile phone addiction: a concurrent study. International Journal of Pharmaceutical Investigation, 2017. Vol. 7, pp. 125–131. DOI:10.4103/jphi.JPHI_56_17
- Pinho C., Franco M., Mendes L. Application of innovation diffusion theory to the E-learning process: Higher education context. Education and Information Technologies, 2021. Vol. 26, no. 1, pp. 421–440. DOI:1007/s10639-020-10269-2
- Ploton P., Mortier F., Réjou-Méchain M., Barbier N., Picard N., Rossi V., Pélissier R. Spatial validation reveals poor predictive performance of large-scale ecological mapping models. Nature communications, 2020. Vol. 11, no. 1, pp. 4540–4562.
- Posit team RStudio: Integrated Development Environment for R. Posit Software, PBC, Boston: MA, 2023.
- R Core Team. R: A language and environment for statistical computing. Vienna, Austria: R Foundation for Statistical Computing,
- Reuver M., Bouwman H. Dealing with self-report bias in mobile Internet acceptance and usage studies. Information & Management, 2015. 52, no. 3, pp. 287–294.
- Rissel C., Badcock P.B., Smith A.M., Richters J., De Visser R.O., Grulich A.E., Simpson J.M. Heterosexual experience and recent heterosexual encounters among Australian adults: the Second Australian Study of Health and Relationships. Sexual Health, 2014. Vol. 11, no. 5, pp. 416–426.
- Rozgonjuk D., Kattago M., Täht K. Social media use in lectures mediates the relationship between procrastination and problematic smartphone use. Computers in Human Behavior, 2018. Vol. 89, pp. 191–198. DOI:1016/j.chb.2018.08.003
- Rozza A., Lombardi G., Rosa M., Casiraghi E., Campadelli P. IDEA: Intrinsic Dimension Estimation Algorithm. In Maino G., Foresti G.L. (Eds.). Image Analysis and Processing. Springer- Verlag, 2011. Vol. 6978, pp. 433–442. DOI:10.1007/978-3-642-24085-0_45
- Sapacz M., Rockman G., Clark J. Are we addicted to our cell phones? Computers in Human Behavior, 2016. Vol. 57, pp. 153–159.
- Saraçli S., Doğan N., Doğan İ. Comparison of hierarchical cluster analysis methods by cophenetic correlation. Journal of inequalities and Applications, 2013. Vol. 1, pp. 1–8.
- Schmiedeberg C., Huyer-May B., Castiglioni L., Johnson M.D. The more or the better? how sex contributes to life satisfaction. Archives of Sexual Behavior, 2017. Vol. 46, no. 2, pp. 465–473.
- Sullivan J.H., Warkentin M., Wallace L. So many ways for assessing outliers: What really works and does it matter? Journal of Business Research, 2021. Vol. 132, pp. 530–543.
- Starrs A.M., Ezeh A.C., Barker G. Accelerate progress–sexual and reproductive health and rights for all: report of the Guttmacher–Lancet Commission. Lancet, 2018. Vol. 391, pp. 2642–2692. DOI:10.1016/S0140-6736(18)30293-9
- Steelman Z.R., Soror A.A. Why do you keep doing that? The biasing effects of mental states on IT continued usage intentions. Computers in Human Behavior, 2017. Vol. 73, pp. 209–223. DOI:1016/j.chb.2017.03.027
- Tenenbaum J.B., De Silva V., Langford J.C. A Global Geometric Framework for Nonlinear Dimensionality Reduction. Science, 2000. Vol. 290, pp. 2319–2323.
- Thomee S., Dellve L., Harenstam A., Hagberg M. Perceived connections between information and communication technology use and mental symptoms among young adults – a qualitative study. BMC Public Health, 2010. Vol. 10, no. 1, pp. 66–82.
- Turel O., Mouttapa M., Donato E. Cyber spaces/social interactions. Preventing problematic internet use through video-based interventions: A theoretical model and empirical test. Behaviour & Information Technology, 2015. Vol. 34, pp. 349–362. DOI:10.1080/0144929X.2014.936041
- Twenge J.M., Sherman R.A., Wells B.E. Changes in American adults’ reported same-sex sexual experiences and attitudes, 1973–2014. Archives of Sexual Behavior, 2016. Vol. 45, no. 7, pp. 1713–1730.
- We Are Social & Hootsuite. (report). Digital 2023. Retrieved June 7, 2023. URL:https://datareportal.com/reports/digital-2023-global-overview-report (Accessed 14.04.2024).
- Wellings K., Johnson A.M. Framing sexual health research: adopting a broader perspective. Lancet, 2013. Vol. 382, pp. 1759–1762. DOI:10.1016/S0140-6736(13)62378-8
- Wellings K., Palmer M.J., Machiyama K., Slaymaker E. Changes in, and factors associated with, frequency of sex in Britain: evidence from three National Surveys of Sexual Attitudes and Lifestyles (Natsal). British Medical Journal, 2019. Vol. 365, pp. l1525–l1542. DOI:10.1136/bmj.l1525
- Yook I.H., Park S.J., Choi M.J., Kim D.-J., Choi I.Y. Factors affecting smartphone usage self-report levels. Studies in Health Technology and Informatics, 2019. Vol. 264, pp. 1937–1938. DOI:3233/shti190722
Information About the Authors
Metrics
Web Views
Whole time: 434
Previous month: 20
Current month: 0
PDF Downloads
Whole time: 149
Previous month: 4
Current month: 0
Total
Whole time: 583
Previous month: 24
Current month: 0